Imagine the following scenario: You are a manager in a consumer bank that offers customers a savings account with a minimum deposit and minimum of six months before cash withdrawals can be made.
However, if a customer is dissatisfied with the account, they can immediately close it and get their money back. Regardless of whether or not they close their account within six months, the sales employee who set it up is still paid a commission.
In such a scenario, an employee could seek to inflate their commission by influencing a customer to open an account in order to be eligible for another product (an accompanying loan, for example) with the proviso that the savings account can be closed, without penalty, immediately thereafter.
Certainly, such situations are not uncommon, and can occur in one form or another, in any organisation. But what if you could predict the occurrence of such ‘bogus’ transactions and take action before they even occur?
The predictive audit
As it turns out, the rapid advancement in technology, and the development of data mining and data analytics techniques over the past decade, have led to the development of a methodology that can allow businesses to predict and prevent such anomalies before they occur.
The predictive audit is a forward-looking approach that examines the validity of transactions before they are executed. It does so by comparing actual transactions to timely normative models, allowing managers to be alerted to potentially problematic transactions before they occur. This gives senior staff the opportunity to investigate and resolve any issues before allowing flagged transactions to go through.
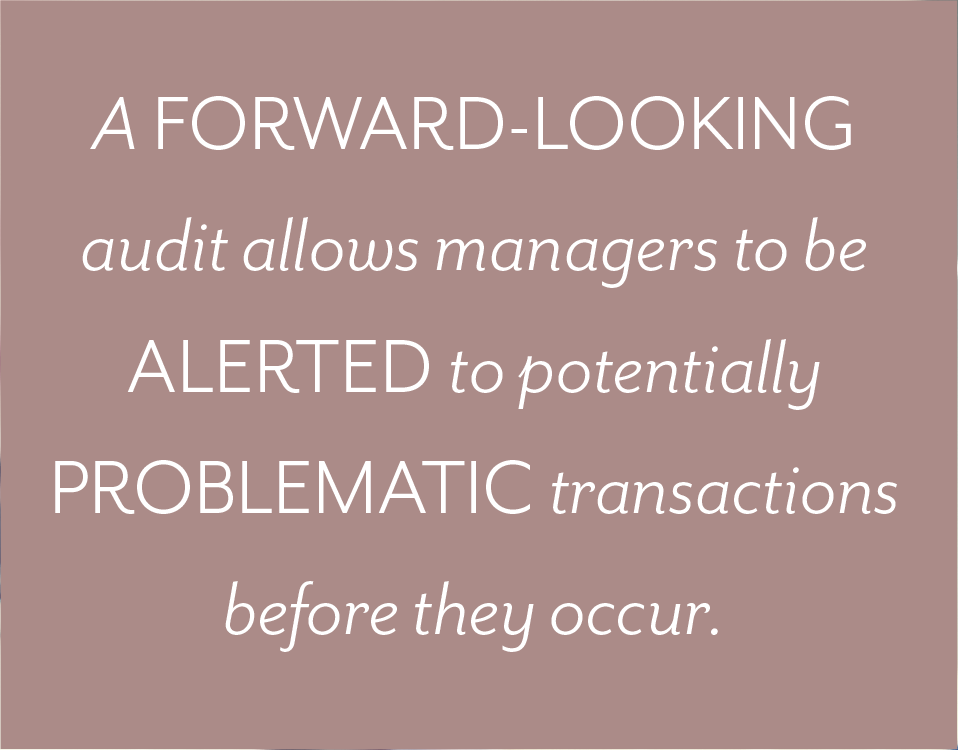
In applying a prognosticative approach, this audit focuses on future events and leverages on technology to develop techniques that predict the likelihood of certain irregularities. In some cases, it can also go one step further to perform a preventive function to avoid the execution of defective transactions.
The predictive audit turns the traditional method on its head. While an audit is traditionally backward-looking and performed on a periodic basis, the predictive audit looks forward and is carried out on a continuous basis.
Embracing the future
One company that has embraced this method is Singapore-headquartered DBS Bank, one of the largest financial services organisations in Asia. At DBS, an internal audit is built around being productive and predictive, and focuses on proactive and preventive risk identification.
In particular, it has employed the methodology to develop data modelling for predictive analysis, cyber intelligence and early IT incident intervention. It now uses advanced analytics to pick up anomalies in trading patterns and to perform credit and fraud analysis by tapping into its vast wealth of data.
Taking its fraud analysis a step further, DBS has correlated disparate data sets to build models identifying the risk profiles of its individual branches. This approach has played a key role in enhancing the bank’s risk management and regulatory compliance.
Making predictions
The predictive model can enhance the control environment of an organisation and also lead to improved feedback mechanisms for auditors. In particular, they can not only examine errors and irregularities that cause particular transactions to be flagged but can also ensure that prompt measures are taken to investigate and resolve them.
Auditors have long used analytical methods to identify relationships between sets of data, and the predictive model builds on this to make specific predictions about certain business outcomes.
Such predictions are now within the reach of companies due to several developments. First, data is now abundant and neatly stored in a digital format. Second, with the adoption enterprise resource planning (ERP) systems and data warehouses, auditors and managers today have an almost unlimited access to data for their prediction models.
Leveraging on these, audit models can be used to make predictions in the following areas: risks (operational, environmental, and black swan events); control trends (especially important since Sarbanes-Oxley); and financial levels and flows. With the evolution of newer and better technologies, the era of real-time auditing and control is upon us. The technologies used can be applied in creative and innovative ways.
For example, in its drive to develop predictive auditing within its organisation, DBS Bank collaborated with the Institute of Infocomm Research in Singapore to develop a data analytics-based solution that relies on a data-driven, learning, risk surveillance model that analyses heterogeneous data to detect and predict risk events. The collaboration was so successful that the project was one of the winners in the IES Prestigious Engineering Awards in 2015.
Today, senior managers and auditors do not just want to verify past activities; they want to predict future events in order to improve control, and to prevent anomalous transactions. The predictive audit model is a rapidly emerging and maturing concept that could realise this vision.
How to create your predictive audit model
Determine your risk profile
Identify the risks your business faces, the potential impact of these and your tolerance for them.
Understand your processes
Clearly identify the specific process you want to develop an auditing model for. This helps clearly define the scope of your project.
Collect data
Predictive analytics relies on the availability of relevant data. It is also crucial that the data collected is cleaned before being used.
Develop and run your model
Various techniques, from basic statistical to sophisticated analytical modelling, can be used in the predictive models.
These should be run multiple times to simulate different scenarios in order to predict various outcomes under different circumstances. It is important to cover as many scenarios as possible to ensure that the model is robust.
Create KPIs
Each result should be compared to a set of pre-defined benchmarks or KPIs. These could be based on existing benchmarks or developed for use with the predictive audit model.
Audit by exception
Deviations from these benchmarks may be indications of anomalies, and should be flagged and investigated. These exceptions are generated in real time, which allows managers to investigate them as they occur.